Unveiling the Challenge of Disagreements: A summary
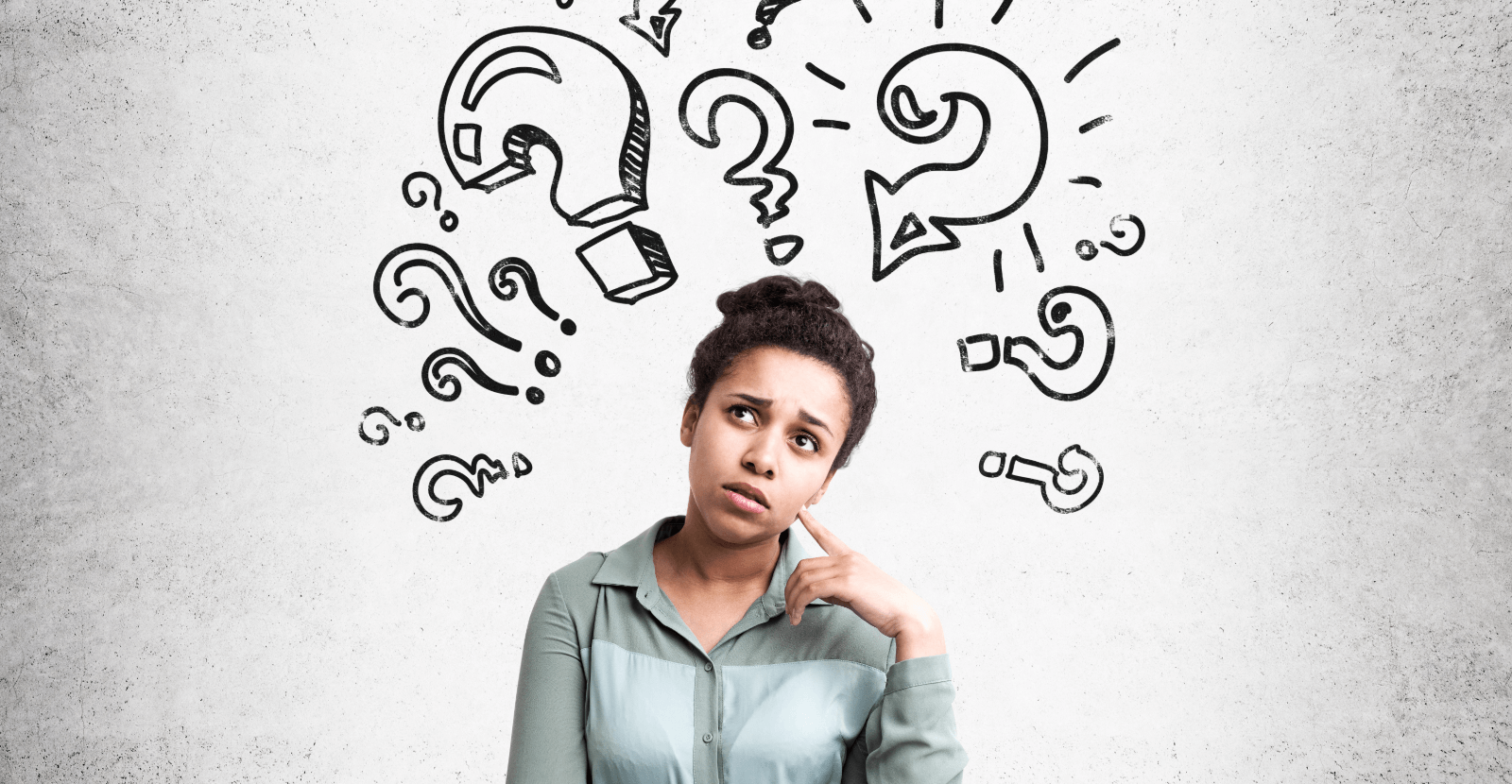
In the expansive landscape of artificial intelligence, the quest for transparency and interpretability has given rise to a myriad of tools collectively known as explainable machine learning (XAI). In this context, a critical issue has emerged—disagreements among these explanations. This blog delves into the intricacies of the disagreement problem, exploring the difficulties faced by practitioners and suggesting potential paths for resolution summarized from the research held by Satyapriya Krishna, Tessa Han, Alex Gu, Javin Pombra, Shahin Jabbari, Zhiwei Steven Wu, and Himabindu Lakkaraju. This research is a collaborative work of the world’s renowned institutes Harvard University, Massachusetts Institute of Technology, Drexel University, and Carnegie Mellon University
The Disagreement Problem
Disagreements among various XAI tools have become a common hurdle in real-world applications, posing a threat to the accuracy and reliability of ML models. This issue becomes particularly acute in critical domains where ML models are deployed. Unfortunately, the absence of a standardized methodology for resolving these disagreements compounds the complexity, making it challenging for practitioners to confidently rely on the decisions made by ML models.
Background: Understanding the XAI Toolbox
To comprehend the disagreement problem, we must first navigate through the two main categories of XAI methods: inherently interpretable models and post hoc explanations. Inherently interpretable models, like Generalized Additive Models (GAMs) and decision trees, offer simplicity but come with a trade-off in model complexity. This trade-off has led to the prevalence of post hoc explanation methods, including popular techniques such as LIME, SHAP, and various gradient-based approaches.
Previous studies have attempted to evaluate the fidelity and stability of these explanations, introducing metrics such as fidelity, stability, consistency, and sparsity. However, as research progressed, the discovery of inconsistencies and vulnerabilities within existing explanation methods, including susceptibility to adversarial attacks, raised concerns about their reliability.
Methodology: Unraveling Disagreements
This study, conducted by Krishna S. and researchers, addressed the disagreement problem through a multifaceted approach:
Semi-Structured Interviews:
Interviews with 25 data scientists revealed that 88% of practitioners utilize multiple explanation methods, with 84% encountering frequent instances of disagreement. Factors contributing to disagreement include different top features, ranking among top features, signs in feature contribution, and relative ordering among features.
Framework for Quantifying Disagreement:
The researchers designed a novel framework to quantitatively measure disagreement using six metrics: feature agreement, rank agreement, sign agreement, signed rank agreement, rank correlation, and pairwise rank agreement. These metrics provide a comprehensive evaluation of disagreement levels.
Empirical Analysis:
Employing four datasets, six popular explanation methods, and various ML models, the researchers conducted an empirical analysis that uncovered trends in disagreement based on model complexity and granularity of data representation (tabular, text, and image). Notably, disagreement tends to increase with model complexity.
Qualitative Study:
A qualitative study explored decisions made by data scientists when facing explanation disagreements. Findings revealed a lack of formal agreement on decision-making, with participants relying on personal heuristics and preferences for certain methods.
Results: Illuminating the Path Forward
The results of this comprehensive study offer valuable insights:
Frequency of Disagreement:
The researchers observed a high occurrence of disagreement among explanation methods, prompting the need for a systematic approach to navigate these disparities.
Heuristics and Preferences:
ML practitioners often rely on personal heuristics and preferences when selecting explanation methods, highlighting the subjective nature of decision-making in the face of disagreement.
Metrics for Quantifying Disagreement:
The introduced framework with six quantitative metrics provides a robust means of assessing and comparing disagreement levels, enhancing our understanding of the complexities involved.
Conclusion and Future Directions
In conclusion, the disagreement problem in XAI demands attention and strategic solutions. The study, conducted by Krishna S. and researchers, not only uncovers the prevalence of disagreement but also introduces a framework for its quantitative measurement. Future research should delve into the root causes of disagreement, propose innovative resolution methods, and establish reliable evaluation metrics.
As we navigate the intricate landscape of XAI, the journey is marked by challenges, discoveries, and the collective effort of practitioners and researchers alike, seeking clarity in the face of disagreement. Regular education and awareness are crucial to equip data scientists with the latest approaches and foster a global community committed to advancing the field of explainable AI.